台湾大学李宏毅机器学习课程
课时介绍
ML Lecture 9-2 Keras Demo 2
课程介绍
李宏毅((Hung-yi Lee)目前任台湾大学电机工程学系和电机资讯学院的助理教授,他曾于2012年获得台湾大学博士学位,并于2013年赴麻省理工学院(MIT)计算机科学和人工智能实验室做访问学者。他的研究方向主要是语义理解、语音识别、机器学习和深度学习)老师的机器学习课程以机器学习和深度学习的基础知识为主,包含了AI领域的各种最新知识和技术点。课程深入简出,通俗易懂,颇受欢迎,非常适合对AI感兴趣的学习者。本课程已经获得李宏毅老师授权。
推荐课程
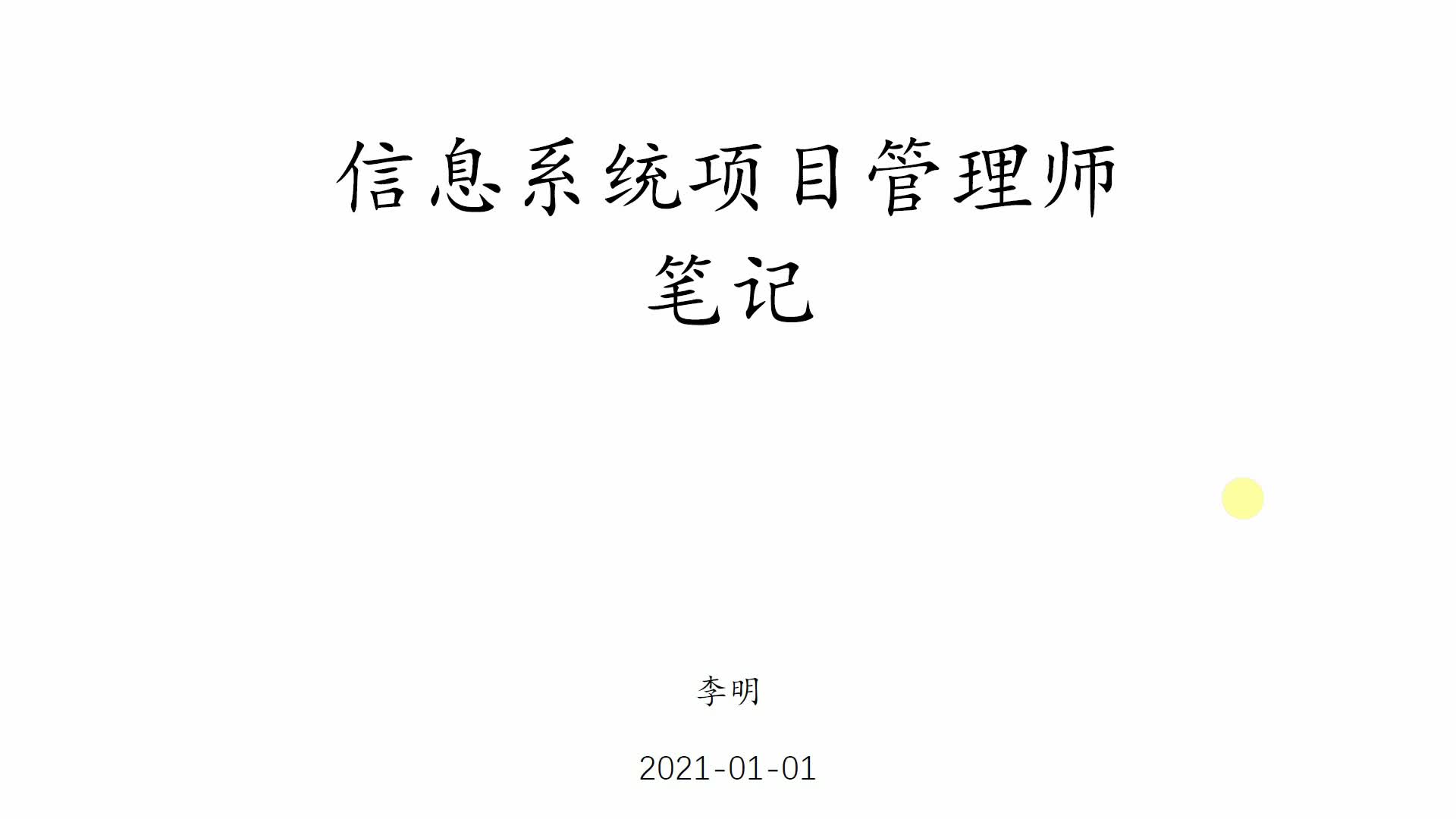
信息系统项目管理师自考笔记
李明 · 710人在学
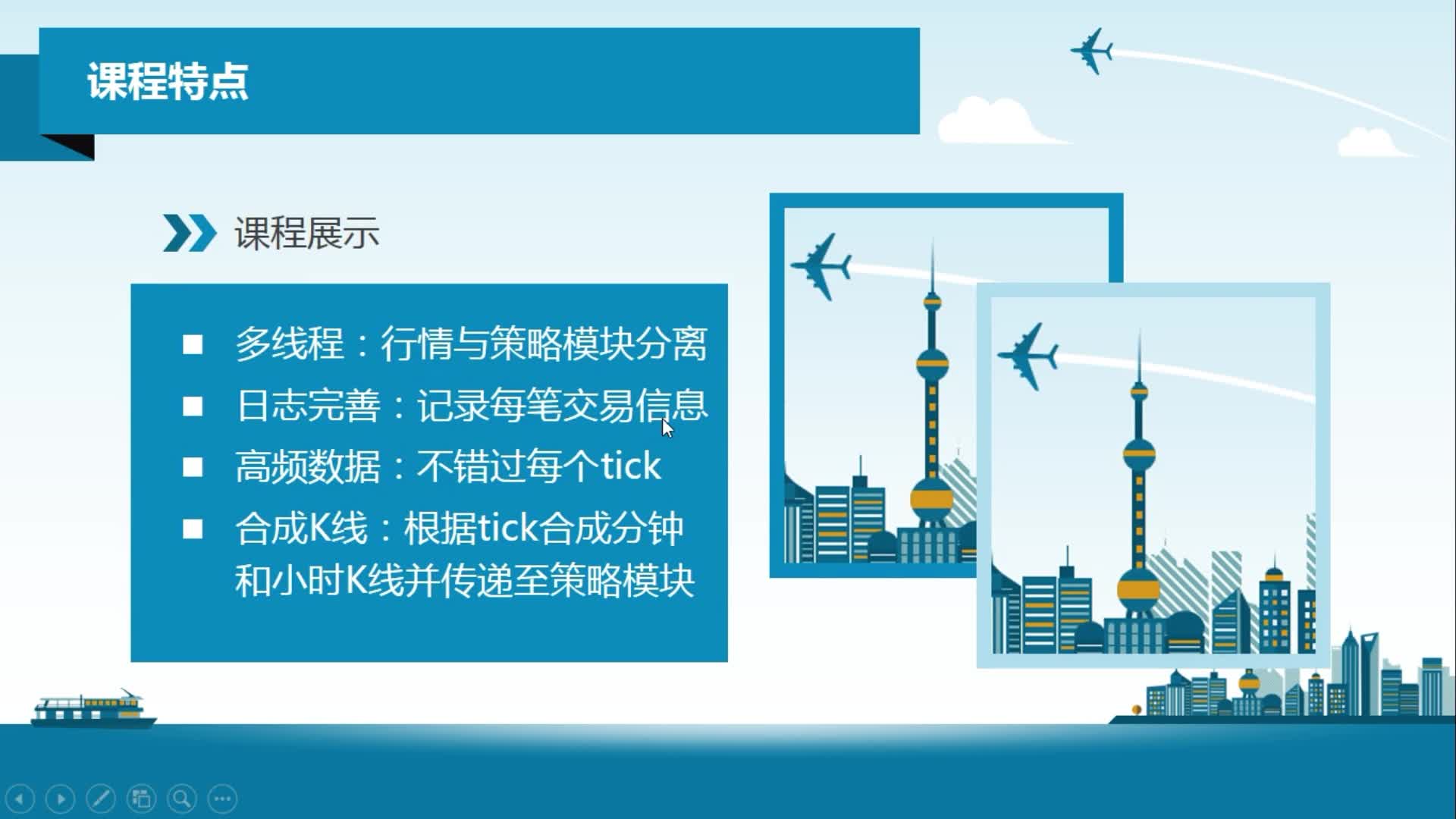
python从0到1:期货量化交易系统(CTP实战,高频及合成K线数据
王先生 · 22071人在学
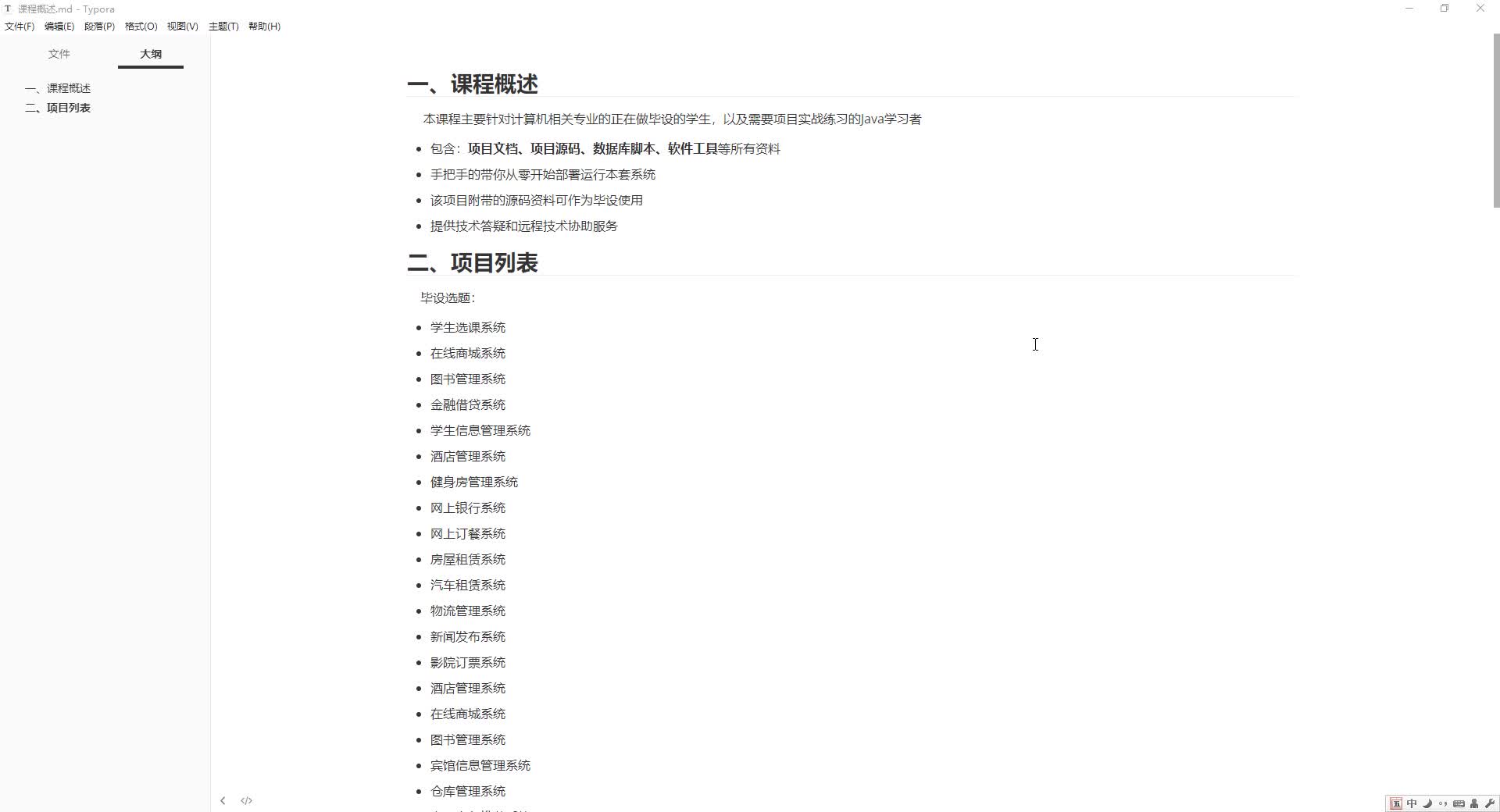
手把手搭建Java超市管理系统【附源码】(毕设)
汤小洋 · 4198人在学
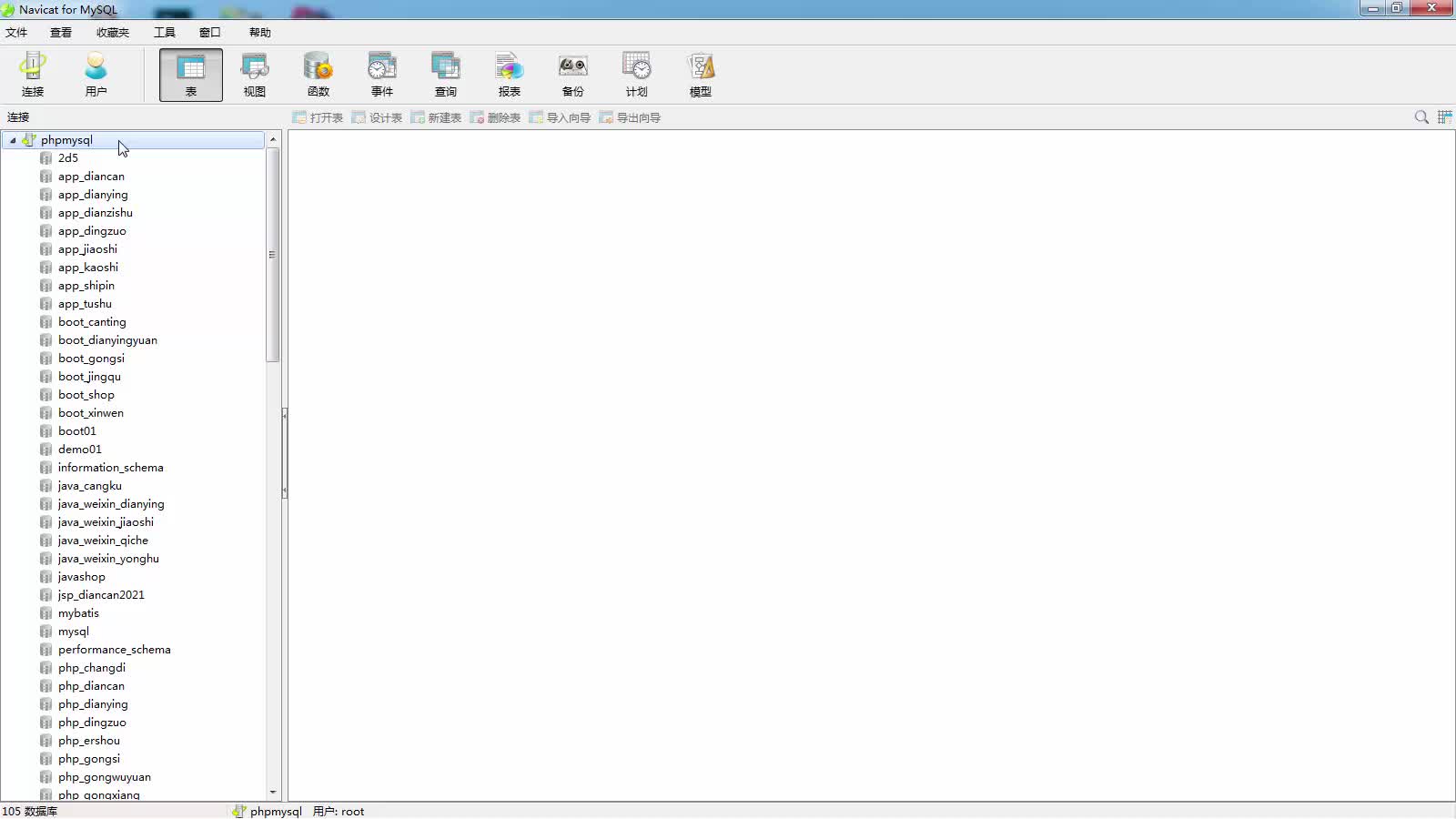
Java毕设springboot外卖点餐系统 毕业设计毕设源码 使用教
黄菊华 · 786人在学
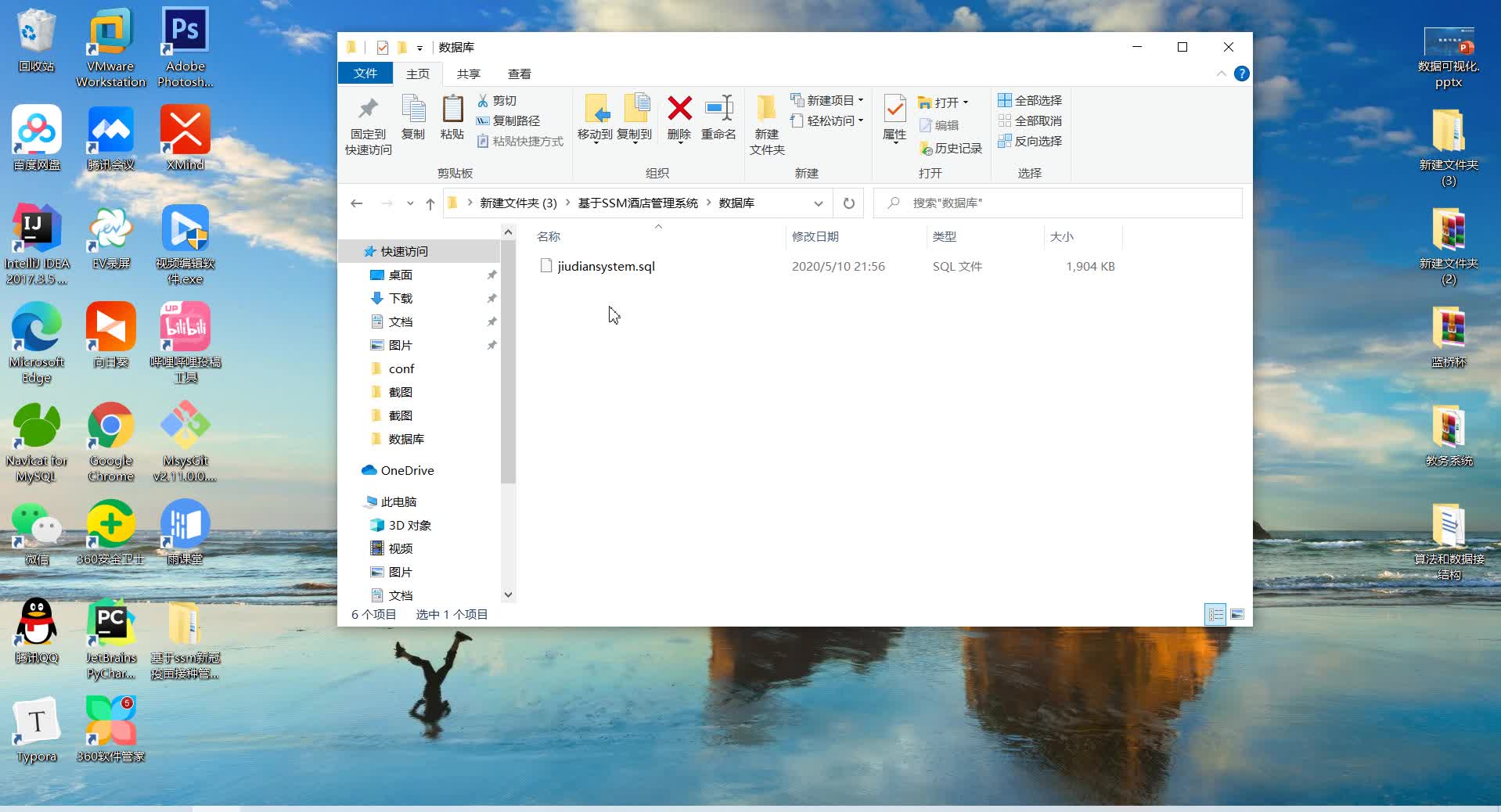
基于SSM酒店管理系统(毕设)
小尼老师 · 837人在学
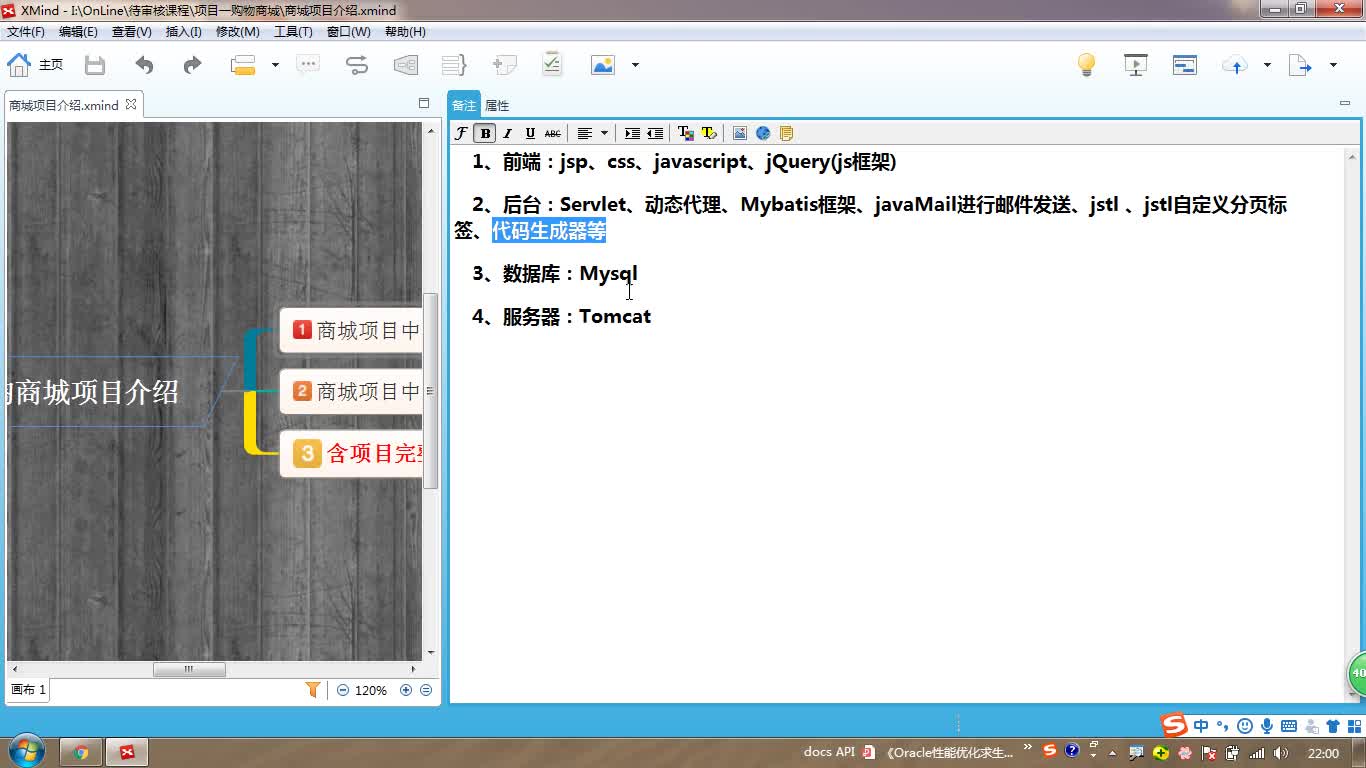
java项目实战之购物商城(java毕业设计)
Long · 5151人在学
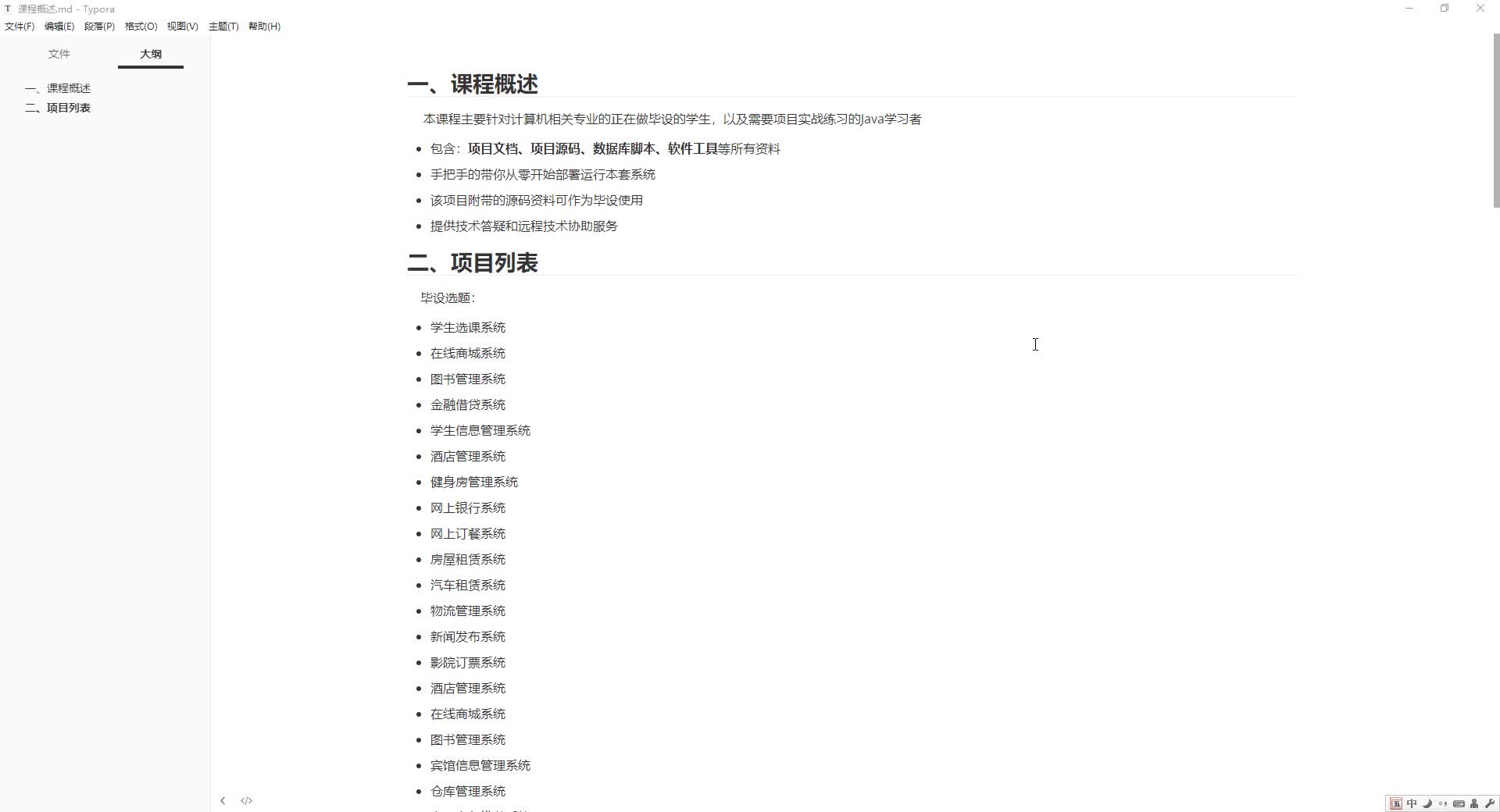
手把手搭建Java求职招聘系统【附源码】(毕设)
汤小洋 · 1504人在学
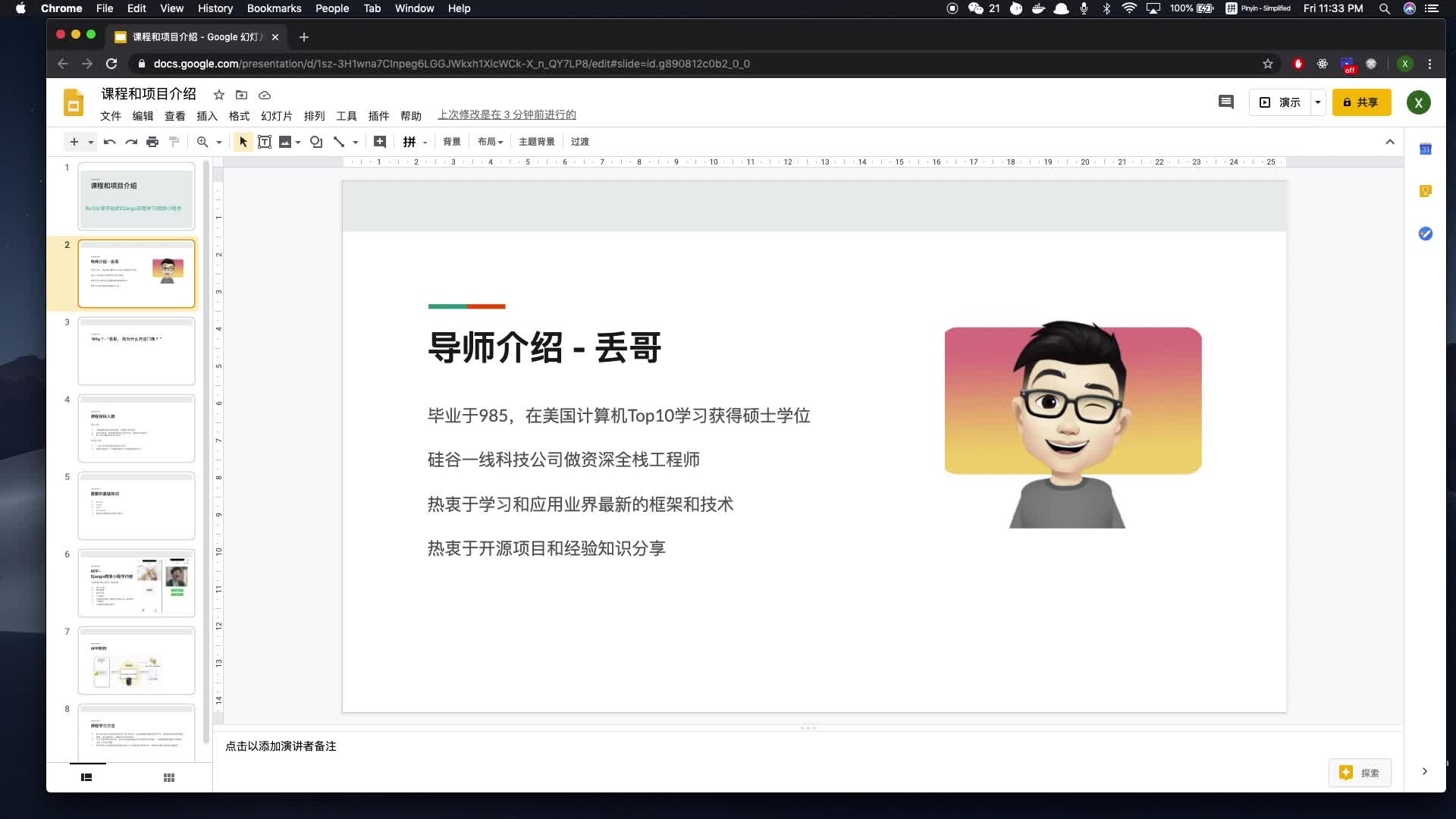
Python Django 深度学习 小程序
钟翔 · 2315人在学
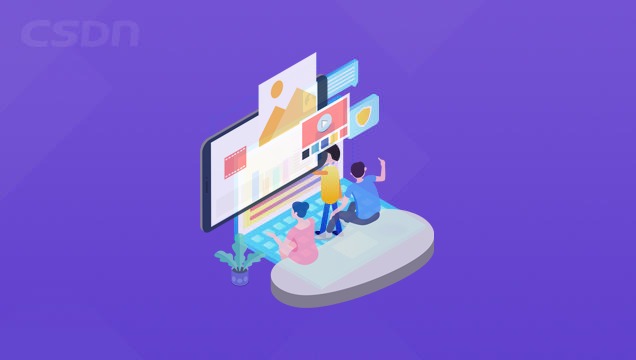
城管局门前三包管理系统+微信小程序(vue+springboot)
赖国荣 · 597人在学
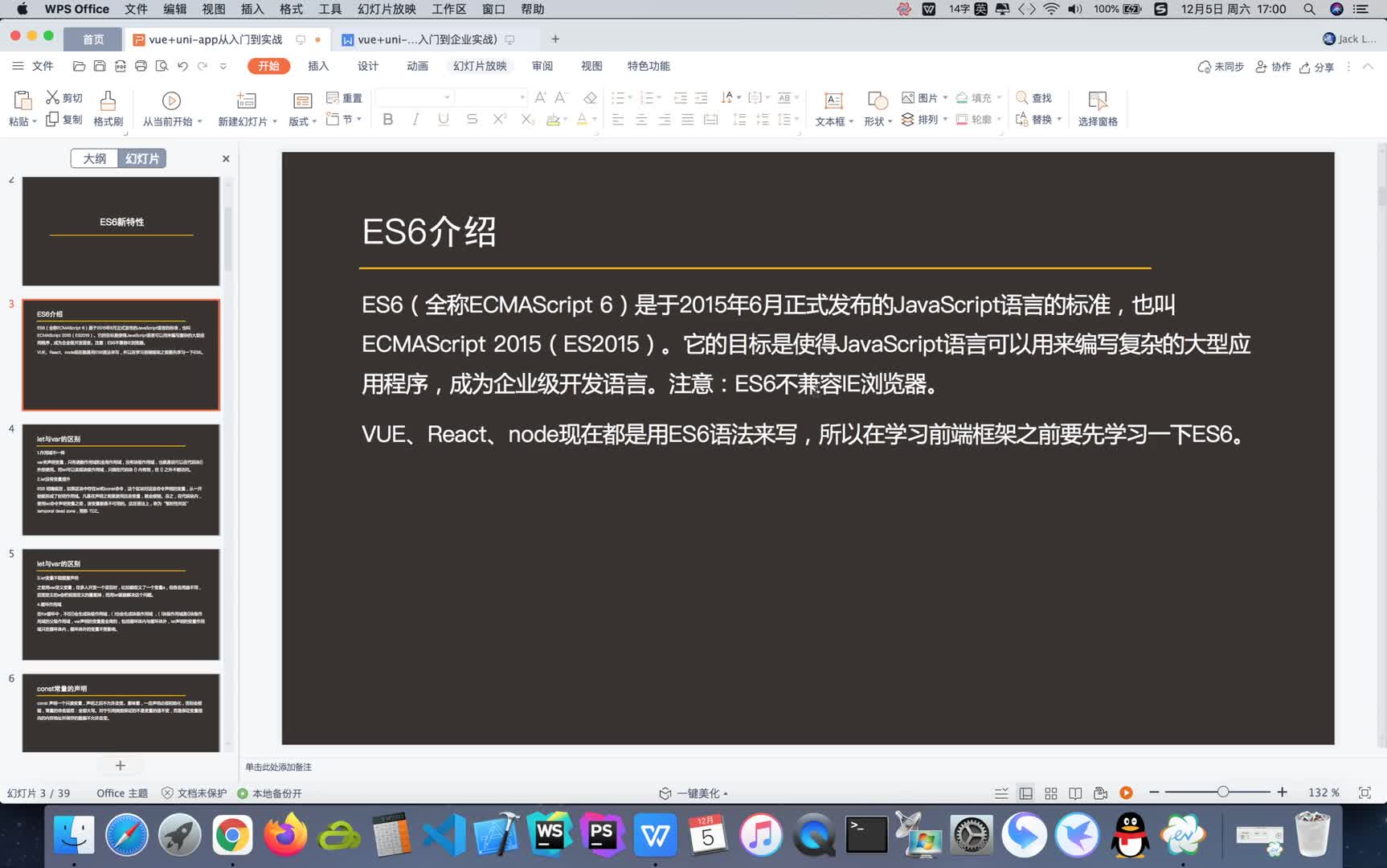
Vue+Uni-app(uniapp)入门与实战+赠送仿美团点餐小程序
李杰 · 3979人在学